ENERGY, POWER & UTILITIES
Predictive Maintenance & Input/Output Optimization for an Oil & Gas Company
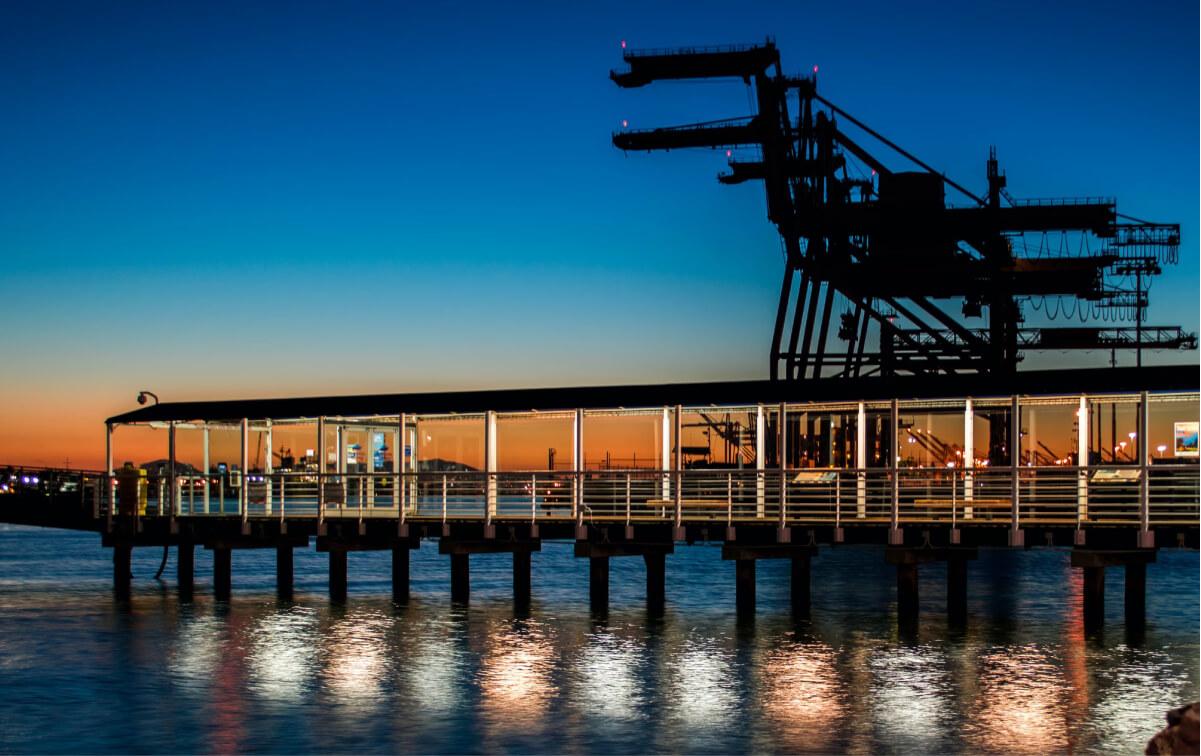
The Challenge
The maintenance engineers of a top oil and gas organization were relying on implicit rules and intuition to identify potential compressor issues, resulting in both human error and unnecessary maintenance costs. Moreover, there was no system for understanding how hundreds of different sensors, implemented by our client for quality control, interacted with one another and with the internal systems to correctly monitor issues.
The Solution
Addo AI developed a predictive maintenance AI engine using the data from hundreds of sensors installed in manufacturing machines and compressors. To build such an engine, a pool of highly skilled Machine Learning Consultants and Machine Learning Engineers were engaged.
Our solution involved building and training a proprietary machine-learning model using data from sensors to detect anomalies in the industrial machines, as well as designing a distributed data architecture — which allowed applications to access data from local and remote databases — to enable real-time predictive analysis.
The AI Engine used techniques to generate reliability reports and health statuses for each machine based on data such as last maintenance date and working status.
AI techniques used
- LSTM-based Encoder-Decoder for multi-sensor anomaly detection
- Recurrent Neural Network (RNN) for the prediction of sensor readings
The Results
- A machine reliability reporting framework was created for anomaly analysis
- Dashboards for real-time asset monitoring of almost 100 sensors were developed, with trigger alerts for potential failures
- A scalable Minimal Viable Product (MVP) was developed on the cloud
Technologies Used
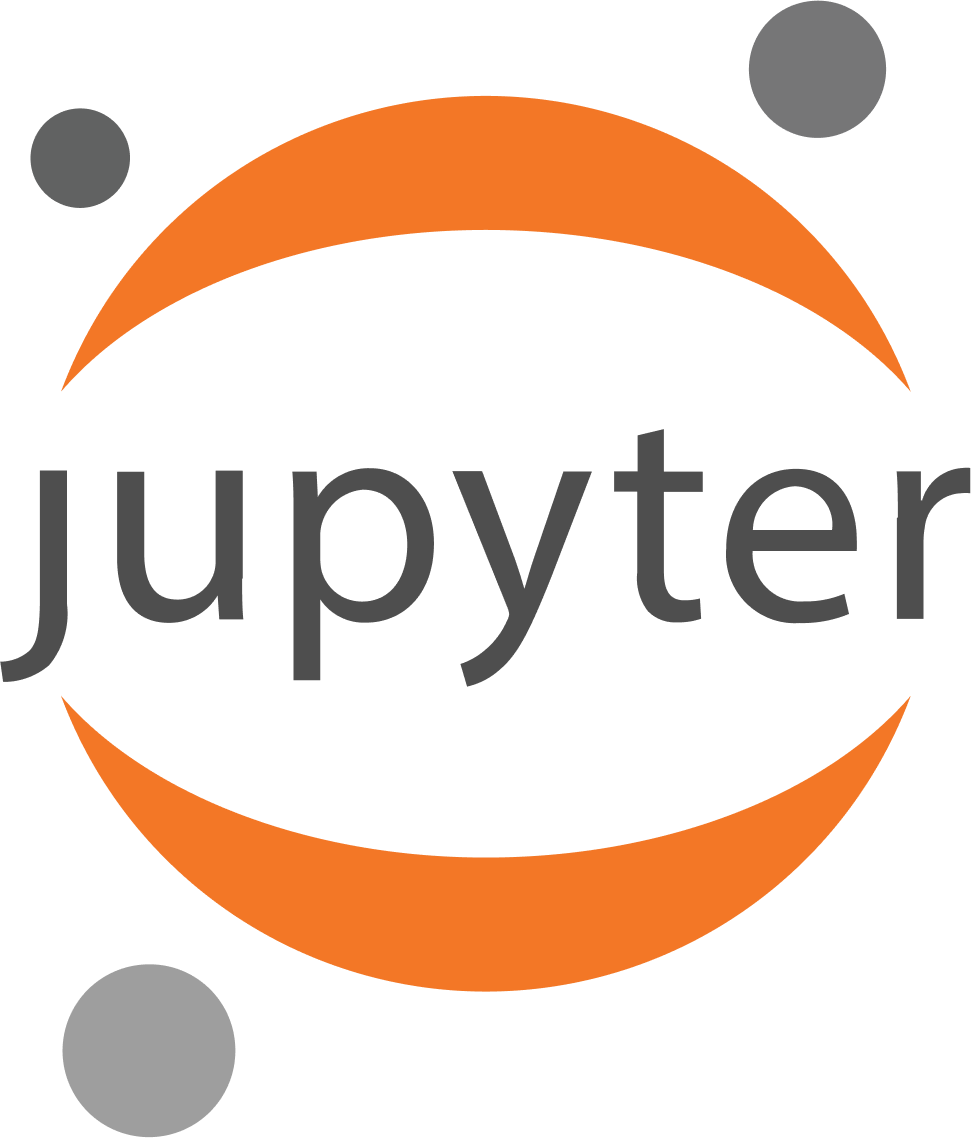
JUPYTER
Impact by the NUMBERS
>100
sensors monitored in real-time
30%
reduction in worker FTE