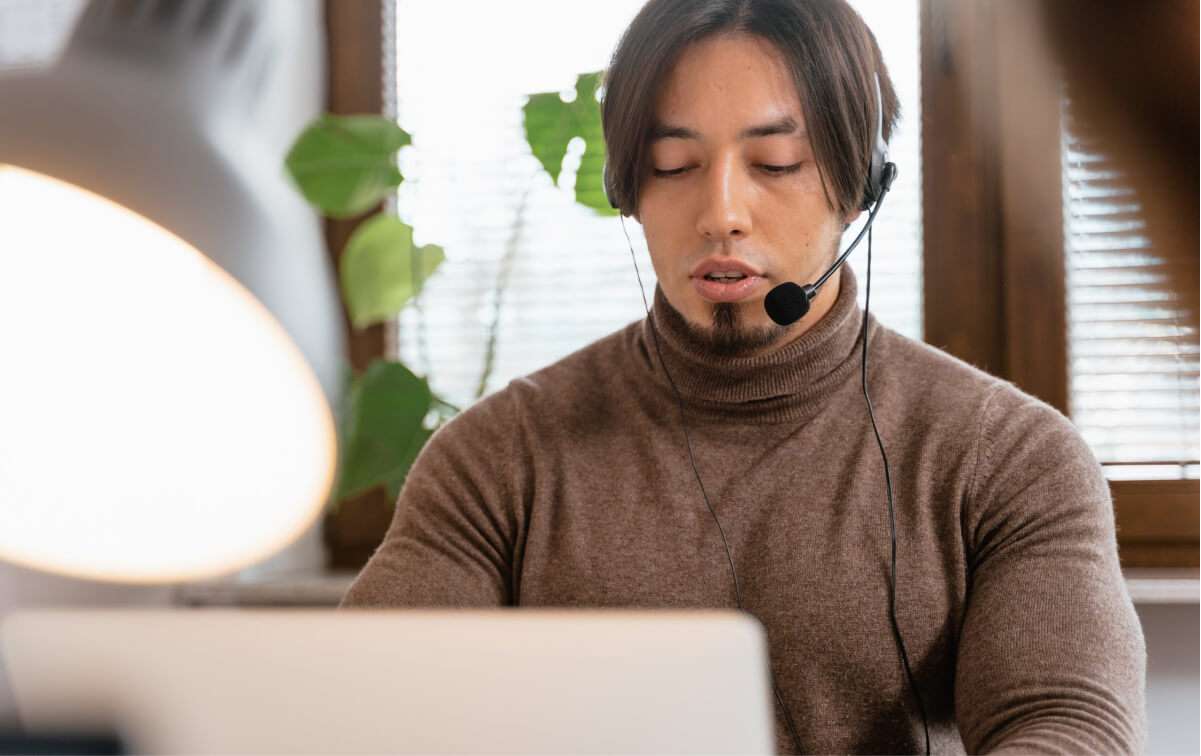
The Challenge
One of Asia’s leading telcos, that receives about 25K+ customer service emails a month, faced challenges in improving customer support efficiency and addressing issues promptly. They required an incident automation platform that utilizes AI/ML technology to speed up incident ticket processing, reduce errors in incident classification, and decrease troubleshooting time for engineers.
The Solution
Addo AI built a customized incident automation platform based on two core AI modules – no-incidents detection & filtering, and root cause localization – with the goal to achieve the following:
- Automatically classify customer emails and incident tickets via agents and direct them to the right engineer.
- Identify the incidents and learn from historic tickets to resolve them faster.
Our approach was three-fold. Firstly, to filter out all the no incidents, secondly, to use the historical data to determine root causes of the incidents and thirdly, to auto-resolve the issues.
We realized that no incidents constitute around 40% of the tickets, so as the very first step, we used state-of-the-art ML and AI techniques, such as Time series analysis, rule base engine and decision tree to filter them out and close them without any human intervention. Once we were left with only the relevant incident events, we used Deep Learning models such as neural networks to determine the top 3 probable root causes of the incidents based on the historic data and allocated them priority scores and confidence levels. Lastly, an automated heal trigger AI Engine was built and integrated with the client system to auto-resolve issues by generating relevant triggers for the hardware/software.
To build this automated incident management platform, a pool of highly skilled Machine Learning Consultants, Machine Learning Engineers, Data Engineers and Integration Engineers were engaged.
The Results
As a result, a significant increase in net promoter scores for customers was observed. Simultaneously, the misallocation of resources and incident resolution time was highly reduced as the engineer’s work for root cause analysis was automated.
ML techniques used:
- Word2Vec and Doc2Vec for Feature Engineering
- Deep Neural Networks
- Ensemble Methods (SVM, Gradient Boosted Trees)
Technologies Used

tensorflow
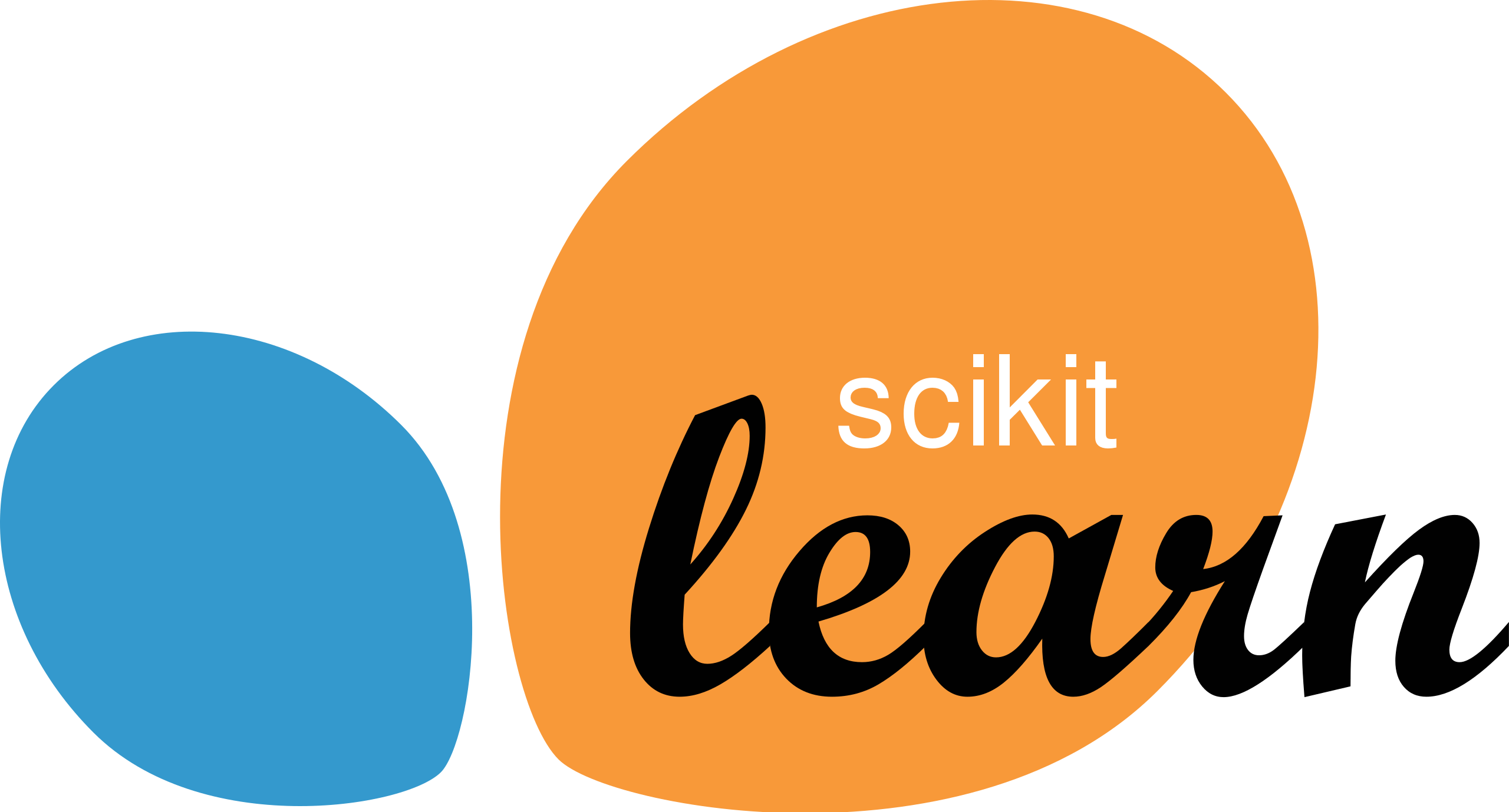
Scikit Learn
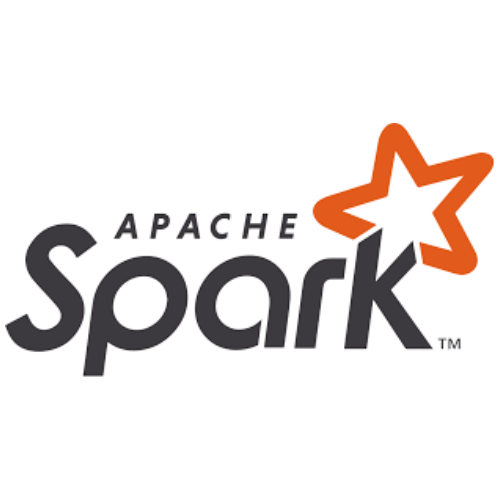
Apache Spark
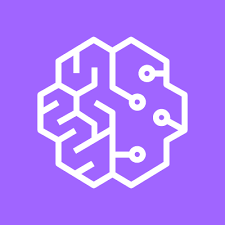
AWS Sage Maker
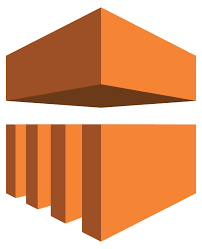
AWS EMR
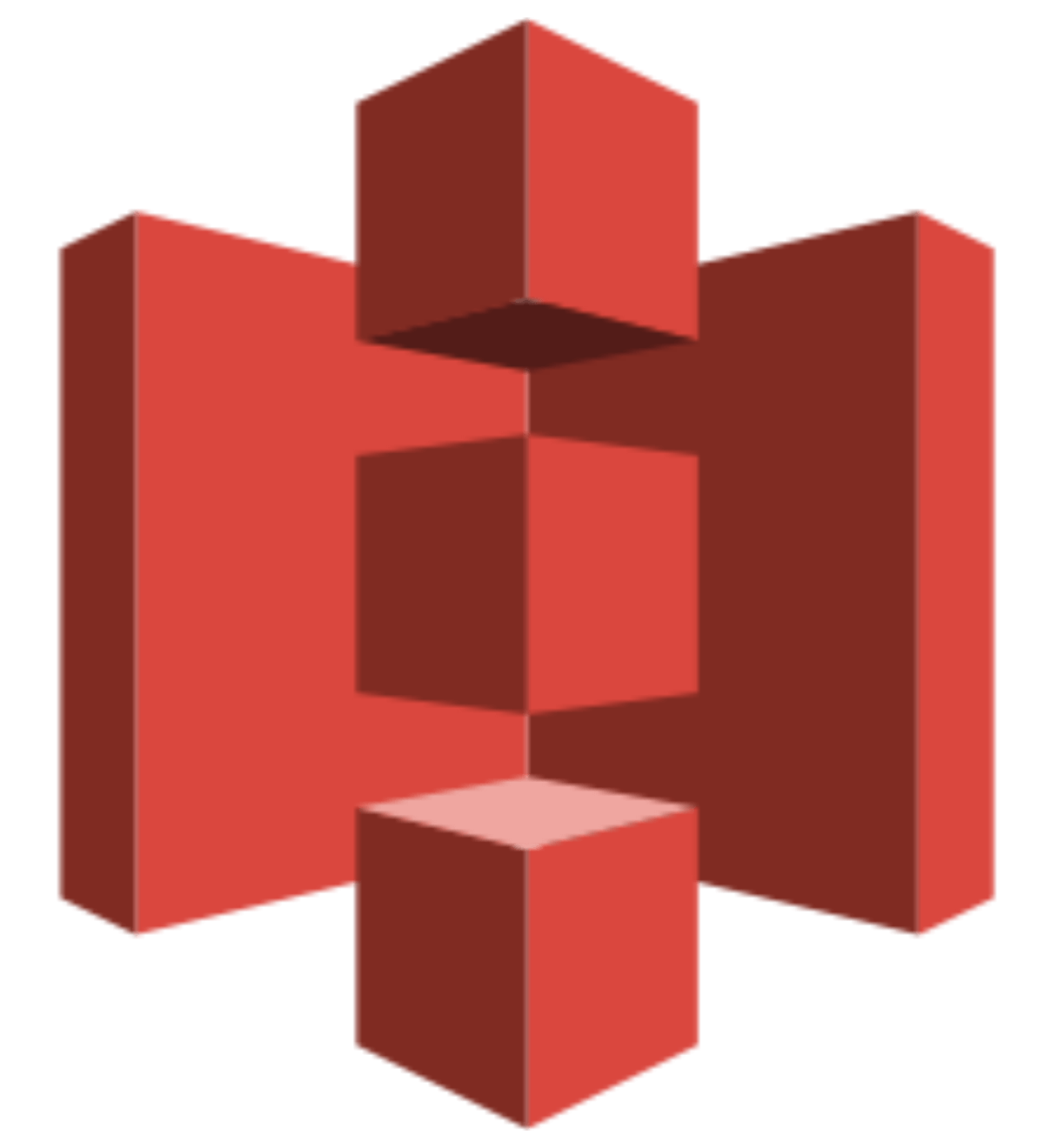
AWS S3 Bucket
Impact by the NUMBERS
40%
Reduction in avg. incident resolution time
20%
Reduction in FTE